Evaluating Multi-Agent Modeller Representations
October 10, 2022
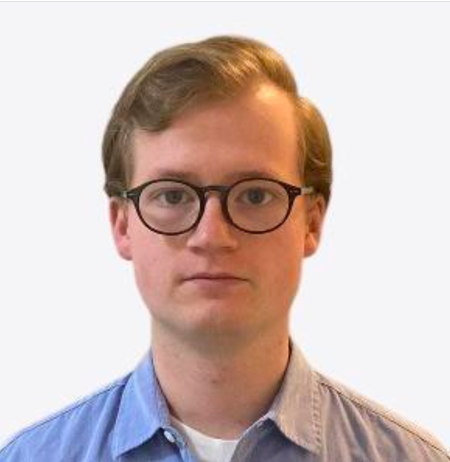
Wednesday, November 2nd at 12pm, TMCB 335
Advisor: Dan Ventura
MS Thesis Defense/PhD Qualifying Process for Jonathan Demke
Abstract:
The way a multi-agent modeller represents an agent not only affects its ability to reason about agents but also the interpretability of its representation space as well as its efficacy on future downstream tasks. We utilize and repurpose metrics from the field of representation learning to specifically analyze and compare multi-agent modellers that build real-valued vector representations of the agents they model. By generating two datasets and analyzing the representations of multiple LSTM- or transformer-based modellers with various embedding sizes, we demonstrate that representation metrics provide a more complete and nuanced picture of a modeller's representation space than an analysis based only on performance. We also provide insights regarding LSTM- and transformer-based representations. Our proposed metrics are general enough to work on a wide variety of modellers and datasets.