Semantically Aligned Sentence-Level Embeddings for Autonomy
July 22, 2019
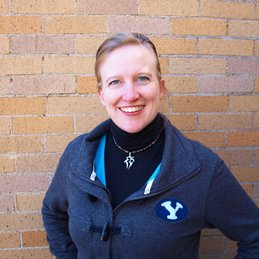
Nancy Fulda
PhD Dissertation Defense
July 31, 2019 at 11:00 am
3350 TMCB
Advisor: David Wingate
Semantically Aligned Sentence-Level Embeddings for Autonomy Nancy Fulda PhD Dissertation Defense
Semantically Aligned Sentence-Level Embeddings for Agent Autonomy and Natural Language Understanding
ABSTRACT:
Many applications of neural linguistic models rely on their use as pre-trained features for downstream tasks such as dialog modeling, machine translation, and question answering. This work presents an alternate paradigm: Rather than treating linguistic embeddings as input features, we treat them as common-sense knowledge repositories that can be queried using simple mathematical operations within the embedding space, without the need for additional training. Because current state-of-the-art embedding models were not optimized for this purpose, this work presents a novel embedding model designed and trained specifically for the purpose of "reasoning in the linguistic domain''.
Our model jointly represents single words, multi-word phrases, and complex sentences in a unified embedding space. To facilitate common-sense reasoning beyond straightforward semantic associations, the embeddings produced by our model exhibit carefully curated properties including analogical coherence and polarity displacement. In other words, rather than training the model on a smorgaspord of tasks and hoping that the resulting embeddings will serve our purposes, we have instead crafted training tasks and placed constraints on the system that are explicitly designed to induce the properties we seek. The resulting embeddings perform competitively on a variety of common-sense and semantic evaluation tasks including analogical reasoning, the Semantic Textual Similarity benchmark, and SemEval 2013, and outperforms state-of-the-art models on two key semantic discernment tasks introduced in Chapter 8.
The ultimate goal of this research is to empower agents to reason about low-level behaviors in order to fulfill abstract natural language instructions in an autonomous fashion. An agent equipped with an embedding space of sufficient caliber could potentially reason about new situations based on their similarity to past experience, facilitating knowledge transfer and one-shot learning. As our embedding model continues to improve, we hope to see these and other abilities become a reality.