Weekly Seminar: Nathaniel Bennett
October 24, 2024
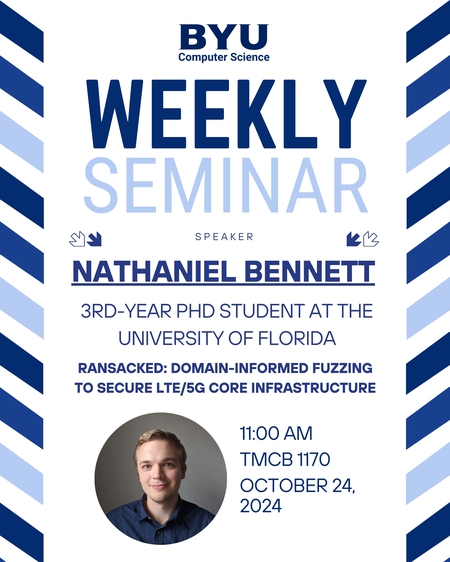
Where: TMCB 1170
When: October 24, 2024, 11am
Come meet Nathaniel Bennett, BYU CS grad and current PhD student at University of Florida!
Bio: Nathaniel Bennett is a 3rd-year PhD student studying at the University of Florida. He received his Bachelors of Science in Computer Science at BYU, and is a current recipient of the NSF Graduate Research Fellowship.
His research focuses include security and privacy in cellular networks and dynamic program analysis. In his free time, Nathaniel enjoys camping and hiking with his wife and 1-year-old daughter.
Title: RANsacked: Domain-Informed Fuzzing to Secure LTE/5G Core Infrastructure
Abstract: Cellular network infrastructure serves as the backbone of modern mobile wireless communication. As such, cellular cores must be proactively secured against external threats to ensure reliable service. Compromised base station attacks against the core are a rising threat to cellular networks, while user device inputs have long been considered as an attack vector; despite this, few techniques exist to comprehensively test RAN-Core interfaces against malicious input. In this talk, we'll explore the technique of fuzz testing and its more recent applications to network-connected applications. We'll then focus specifically on the domain of cellular networks and highlight some of the challenges with fuzzing cellular infrastructure that has hampered the application of current fuzzing approaches. Our research, which devises a fuzzing framework that performantly fuzzes cellular interfaces accessible from a base station or user device, overcomes several of these challenges in fuzzing LTE/5G network components. We also find our efforts in the cellular domain yield cross-domain applications. For instance, we develop and release a tool (ASNfuzzgen) that compiles arbitrary ASN.1 specifications into structure-aware fuzzing modules, thereby facilitating effective fuzzing exploration of protocols across cellular, automotive, space and industrial control systems. We evaluate our approaches against seven open-source and commercial cores and discover 119 vulnerabilities, with 93 CVEs assigned. Our results reveal common implementation mistakes across several cores that lead to vulnerabilities, and the successful coordination of patches for these vulnerabilities across several vendors demonstrates the practical impact ASNfuzzgen has on hardening cellular deployments.
Donuts will be served.