Weekly Seminar: Shandian Zhe
March 08, 2024
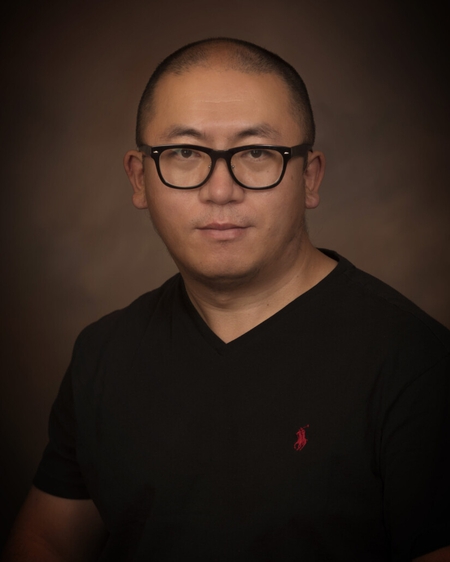
Where: TMCB 1170
When: February 29th @11am
Talk Title: Multi-fidelity Learning and Active Learning for Scientific Machine Learning
Abstract: Multi-fidelity learning involves using training examples at different fidelities or resolutions. High-fidelity examples are of high-quality but often are much more costly to collect than inaccurate, low-fidelity examples. How to retrieve and leverage examples at multiple fidelities is the key to reduce the learning cost while maximizing the efficiency. This talk will introduce our recent work in multi-fidelity learning and active learning for scientific machine learning. Physical simulation is a central task in science and engineering domains. However, traditional numerical methods are known to be computationally costly and lack generalizability across different problems. Learning data-driven surrogate models presents a promising strategy for cost reduction. I will discuss three multi-fidelity, multi-resolution active learning approaches designed to dynamically acquire simulation examples and their fidelities, enhancing surrogate learning performance while minimizing data acquisition costs. I will demonstrate the efficacy of these methods through applications in standard benchmarks of physical simulation and topology structure optimization. Furthermore, I will introduce an infinite fidelity surrogate learning framework, which extends the traditional finite fidelity space to an infinite continuous space. This framework offers exciting possibilities for advancing scientific machine learning by enabling more comprehensive representations of complex systems and phenomena.
Bio: Dr. Shandian Zhe is an Assistant Professor at the Kahlert School of Computing, University of Utah. His research focuses on probabilistic machine learning, AI for science, and complex structured data analysis. Dr. Zhe's work encompasses a range of topics including physics-informed neural networks, operator learning, Gaussian process, governing PDE discovery, tensor decomposition, and high-order interaction event analysis. He has authored over 70 publications, the majority of which have been featured in leading machine learning and data science conferences such as ICML, NeurIPS, AISTATS, and ICDM Dr. Zhe earned his Ph.D. from Purdue University in 2017, during which he was awarded the Google Ph.D. Fellowship in machine learning. He subsequently joined the University of Utah in 2018 and was honored with the NSF CAREER Award in 2021.