Weekly Seminar: Mark Transtrum & Gus Hart
March 31, 2025
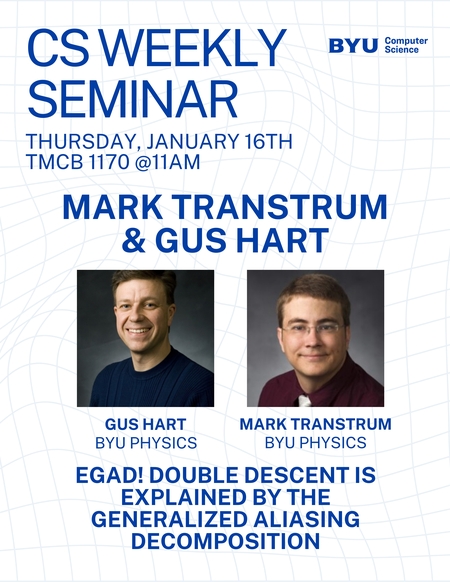
Details
Where: TMCB 1170
When: April 3rd
Speaker: Mark Transtrum & Gus Hart
Talk Title
eGAD! double descent is Explained by the Generalized Aliasing Decomposition
Abstract
A central problem in data science is to use potentially noisy samples of an unknown function to predict values for unseen inputs. Classically, predictive error is understood as a trade-off between bias and variance that balances model simplicity with its ability to fit complex functions. However, over-parameterized models exhibit counterintuitive behaviors, such as “double descent” in which models of increasing complexity exhibit decreasing generalization error. Other models may exhibit more complicated patterns of predictive error with multiple peaks and valleys. Neither double descent nor multiple descent phenomena are well explained by the bias–variance decomposition. I present the generalized aliasing decomposition (GAD) to explain the relationship between predictive performance and model complexity. The GAD decomposes the predictive error into three parts: 1.) model insufficiency, which dominates when the number of parameters is much smaller than the number of data points, 2.) data insufficiency, which dominates when the number of parameters is much greater than the number of data points, and 3.) generalized aliasing, which dominates between these two extremes. I apply the GAD to linear regression problems from machine learning and materials discovery to explain salient features of the generalization curves in the context of the data and model class.
Biography
Mark K. Transtrum received the Ph.D. degree in physics from Cornell University, Ithaca, NY, USA, in 2011. He then studied computational biology as a Postdoctoral Fellow from MD Anderson Cancer Center, Houston, TX, USA. Since 2013, he has been with Brigham Young University, Provo, UT, USA. He is currently an Associate Professor of physics and astronomy. His research works include representations of a variety of complex systems including power systems, systems biology, materials science, and neuroscience.
Gus Hart is a professor in the department of Physics and Astronomy. He came to BYU from Northern Arizona in 2006. He completed a PhD at UC Davis with Barry Klein in 1999 and a postdoctoral appointment at the National Renewable Energy Laboratory in 2001 with Alex Zunger.
Until 2022, Gus' research was computational materials physics where his focus was on alloy modeling, algorithm development, and aflowlib.org. He is the author of enumlib, symlib, and other open source codes. He is the primary developer of the commercial UNCLE cluster expansion code. Google scholar profile.
Since 2022, Gus' research focus has shifted to data science and computational biophysics, with a particular focus on developing AI for bacterial tomograms.