Weekly Seminar: Mckell Woodland
November 07, 2024
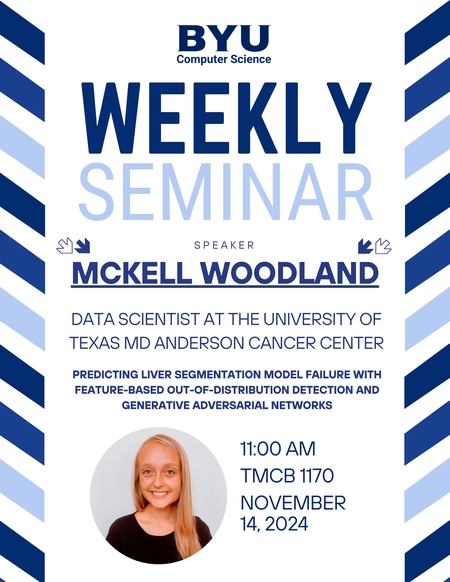
When: November 14th @ 11am
Where: TMCB 1170
Talk Title: Predicting Liver Segmentation Model Failure with Feature-Based Out-of-Distribution Detection and Generative Adversarial Networks
Advanced liver cancer is often treated with radiotherapy, which requires precise liver segmentation. While deep learning models excel at segmentation, they struggle on image attributes not seen during training. To ensure quality care for all patients, my research focuses on automated, scalable, and interpretable solutions for detecting liver segmentation model failures. In this talk, I will first present accurate and scalable solutions that utilize model features extracted at inference. I will then introduce generative modeling for the localization of novel information, an approach that integrates interpretability into the detection pipeline.
Dr. McKell Woodland is a Data Scientist at The University of Texas MD Anderson Cancer Center. She has her Ph.D. in Computer Science from Rice University and a B.S. in Applied Mathematics from Brigham Young University. Dr. Woodland's research focuses on out-of-distribution detection for medical imaging, with an emphasis on the safe integration of deep learning technologies into cancer treatment. She has published her work in venues such as the Machine Learning for Biomedical Imaging Journal and the Medical Image Computing and Computer Assisted Intervention Conference, where she won "Best Spotlight Paper" for her work on out-of-distribution detection.