Weekly Seminar: Taylor Killian
May 08, 2025
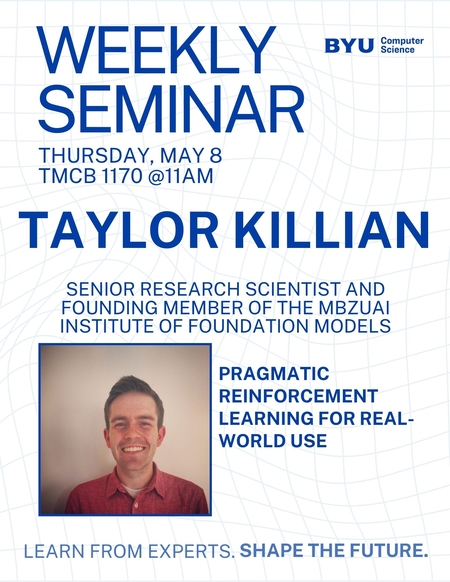
Title: “Pragmatic Reinforcement Learning for Real-World Use”
Abstract: In order to develop practical machine learning aided technology for the benefit of human users, it is critical to anchor scientific research and development by the intended real-world use cases. In this talk, I introduce specific modeling decisions that can be made to develop actionable insights from sequentially observed healthcare data as well as in the development of robust self-driving behaviors via large-scale self-play. By leveraging the inherent structure of the Reinforcement Learning paradigm, we effectively identify behaviors to avoid while also allowing for the development of emergent capabilities. Together, these advances serve to establish a strong foundation from which continued technological progress will be made, several directions that I will outline as a hopeful conclusion of my talk.
Bio:
Taylor is a Senior Research Scientist and Founding Member of the MBZUAI Institute of Foundation Models, Silicon Valley where he co-leads Reinforcement Learning research in both fundamental directions and applied to large language models. Prior to this, he was a Postdoctoral Research Scientist within Apple's Special Projects Group following the completion of a PhD in Computer Science at University of Toronto. His research broadly investigates novel applications of Reinforcement Learning to assist sequential decision making in safety-critical domains. In particular, his interests center on the development of efficient personalized decision support tools that generalize beyond the environment they were trained in, robust to sources of uncertainty such as distribution shift, covariate mismatch, and missing data. Taylor has prior degrees in Computational Science and Engineering (M.Eng, Harvard University) as well as Mathematics (B.S., Brigham Young University) that he has used in prior research positions investigating the RF scheduling algorithms, sensor placement and in the modeling of fluid phenomenon.